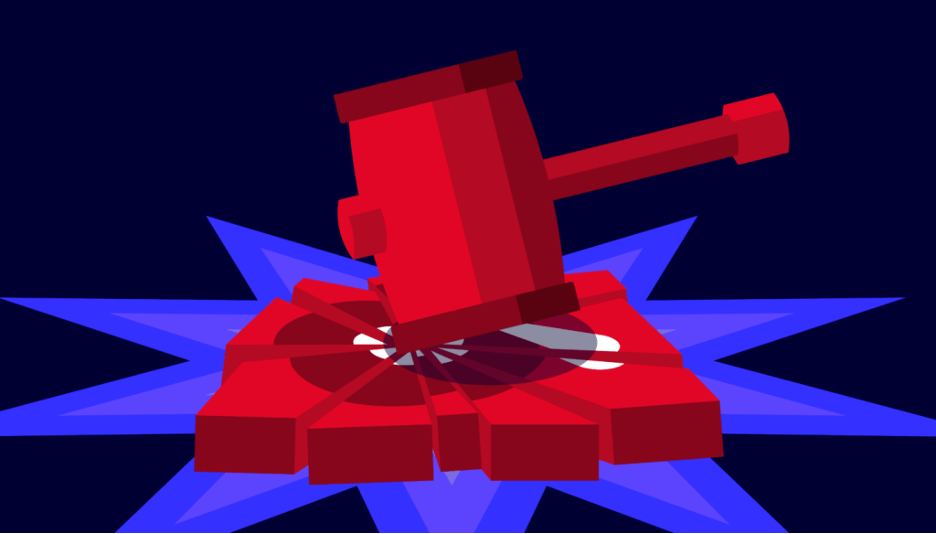
Everyone’s chasing the next breakthrough in AI, pouring money into bigger models and faster chips. But there’s one innovation killer no one’s talking about, and it isn’t compute limits—it’s vendor lock-in.
While you’re optimizing your algorithms, your infrastructure is quietly draining your budget and tying your roadmap to someone else’s agenda. Open cloud providers help you create an ecosystem where data flows freely, innovation isn’t throttled, and every component works harmoniously to drive progress. Yet, for many organizations, vendor lock-in with hyperscalers costs more than just dollars: it comes at the expense of the freedom to innovate on your own terms.
Today, I’m talking through how AI organizations end up locked in with hyperscalers and how to avoid that trap.
Download the ebook
Struggling to keep AI storage costs under control? Download our free ebook to discover how to optimize cloud storage for AI workloads—without compromising performance.
The three pillars of AI infrastructure
At its heart, AI infrastructure rests on three essential pillars:
- Compute: The engines powering your models and training processes.
- Data management: The systems that capture, store, and organize the massive volumes of data your projects depend on.
- Integration and flexibility: The ability to move data seamlessly between various platforms and cloud environments without being tied to a single provider.
When any of these pillars are compromised by vendor lock-in, the consequences are immediate and costly:
- Can you freely move workloads between environments?
- Are you paying premium prices for basic data transfers?
- Is your team spending more time managing infrastructure than building innovative solutions?
These challenges directly hinder your team’s ability to deliver the AI breakthroughs your organization expects.
Understanding vendor lock-in
Vendor lock-in occurs when an organization becomes overly dependent on a single vendor’s products or services, making it difficult—or costly—to switch to alternative solutions. This dependency can manifest in several ways:
- Proprietary technologies: When a vendor’s system uses exclusive formats or interfaces, integrating new tools becomes challenging.
- Complex pricing: Long-term agreements with rigid terms and hidden fees may restrict flexibility and force you to absorb unexpected costs.
- Ecosystem dependence: Relying on one provider’s suite of services can limit your ability to adopt innovative, best-of-breed solutions from other vendors.
In practice, vendor lock-in can hurt innovation by restricting your options, slowing down the pace at which you can adopt new technologies, and diverting resources to manage and maintain a closed system rather than driving creative breakthroughs.
The hidden costs of vendor lock-in
Imagine scaling your AI project only to discover, as Decart did, that egress fees essentially hold your data hostage. Their team needed to train models across multiple GPU clusters simultaneously—a scenario that would have incurred crippling costs with their previous provider. Or consider Grass Network, who found their ability to serve Fortune 1000 clients fundamentally undermined by their cloud vendor’s pricing structure, with egress and deletion fees that made their business model unsustainable at scale.
The pattern is clear: Organizations trapped in vendor-locked systems end up diverting precious resources—both financial and human—away from innovation and toward infrastructure management. This results in delayed training cycles, slower model iterations, and missed market opportunities as engineering talent gets consumed by working around limitations rather than building competitive advantages.
A holistic look at open AI infrastructure
While compute power and advanced analytics often grab headlines, the true strength of an AI system lies in the seamless integration of all its components. An open AI infrastructure can deliver:
- Enhanced agility: With a flexible, multi-cloud approach, you can rapidly adopt new tools and technologies without being bound by a single provider’s limitations.
- Optimized performance: When data flows effortlessly between compute clusters, storage systems, and analytics platforms, every part of your infrastructure can perform at its peak.
- Cost efficiency: Transparent pricing and predictable billing ensure that hidden fees—like those associated with storage tiers and egress—don’t eat into your budget.
- Future-proofing: By avoiding proprietary ecosystems, you build an infrastructure that is resilient and adaptable, giving you the freedom to experiment and innovate.
Rethinking storage as the strategic backbone
Among all the components, storage plays a uniquely critical role—it’s the circulatory system that keeps your data moving throughout the AI workflow. The right storage foundation doesn’t just warehouse data; it becomes a strategic asset that enables multi-cloud workflows, maintains cost predictability, and prevents vendor lock-in by allowing seamless integration with different compute engines, GPU clusters, and software platforms. Forward-thinking organizations are increasingly viewing storage not as a commodity, but as the linchpin of AI infrastructure strategy.
Backblaze B2: A foundation for multi cloud storage
Within this framework, Backblaze B2 serves as a robust storage foundation that transforms how AI teams approach multi-cloud workflows. Rather than trying to be everything to everyone, B2 Cloud Storage focuses exclusively on being the best at what matters most for your data: performance, scalability, and predictability.
It effortlessly scales from terabytes to petabytes, accommodating everything from raw training data to archived model outputs without performance degradation. S3 compatibility means it integrates easily with virtually any AI pipeline or tool. Perhaps most importantly, it keeps costs transparent and predictable with straightforward pricing that eliminates the “sticker shock” that plagues many AI projects.
A reliable, independent storage layer doesn’t just help you sidestep the pitfalls of vendor lock-in—it fundamentally changes how your team approaches innovation by removing the technical and financial barriers that traditionally constrain experimentation.
See the open cloud in action
Your company’s data is a powerful resource—learn how to harness it with an AI agent designed to generate meaningful insights. In this deep dive, Backblaze’s Pat Patterson and Jeronimo De Leon will demonstrate how to build an AI agent that can query, analyze, and generate insights from company-specific data—all powered by cost-efficient, scalable cloud storage.
Charting a new course for innovation
Breaking free from vendor lock-in isn’t merely about cutting costs—it’s about reclaiming control over your entire AI infrastructure and accelerating your path to results. When every component, from compute to storage and integration, is designed to be open and flexible, your organization gains the freedom to experiment, iterate, and push the boundaries of what’s possible.
The most successful AI teams we’ve observed are those building on strong, multi-cloud-friendly foundations where data flows without friction or penalty. They’re the ones asking tough questions about their infrastructure choices today to ensure maximum flexibility tomorrow.